posted on Nov. 12: Redman lecturer explores challenges of Human Genome Project Nov. 12, 13
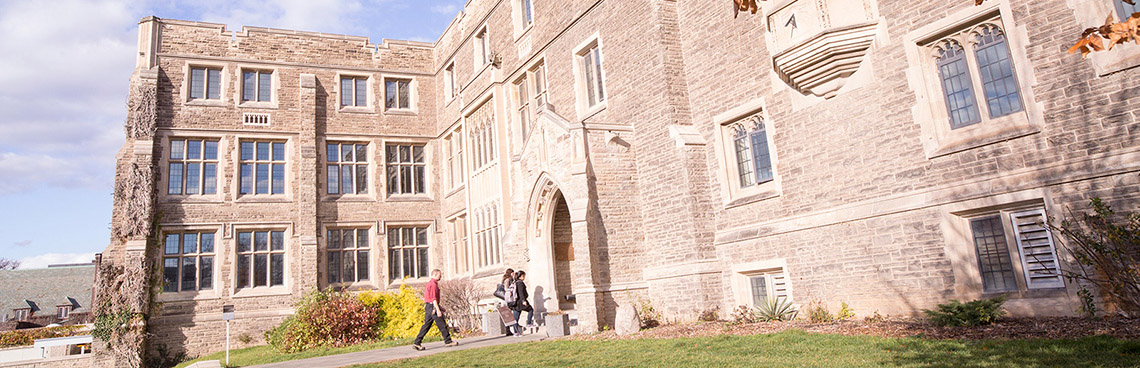
[img_inline align=”right” src=”http://padnws01.mcmaster.ca/images/Haussler.David.Redmanfinal.jpg”]A leading scientist involved in the area of analysis of the human genome using biology, computer science and mathematics will deliver the 2001 Redman Lectures tonight and tomorrow on campus.
This year's Redman lecturer, David Haussler, holds a University of California Presidential Chair in computer science at the
Santa Cruz (UCSC) campus and is director of the Centre for Biomolecular Science & Engineering. He is also a fellow of the American Association for Artificial Intelligence.
Haussler's first lecture, Assembly of the Public Working Draft of the Human Genome, explores the initial public working draft of the
human genome, the challenges
faced in producing the work and the
significance of what was found. This lecture takes place tonight,
Nov. 12 at 8 p.m. in Health Sciences Centre, Room 1A1.
The second lecture, Exploring the Human Genome, provides a tour of the Web browser at UCSC and demonstrates the rich
variety of data mapped to the genome sequence. Haussler will also show comparisons of the human genome to the genomes of
other organisms and discuss how comparative genomics can help us decode important features in our own genome.
This lecture
takes place Tuesday, Nov. 13 at 8 p.m. in Health Sciences Centre, Room 1A1.
Haussler will give an additional special lecture tomorrow, Nov. 13, from 3:30 p.m. to 4:30 p.m. in Hamilton Hall, Room 110, describing his own work in greater detail.
This talk, Hidden Markov Models in Bioinformatics, will focus on how hidden Markov models (HMMs) were initially developed for applications in
speech recognition, but in the last seven years have found many
applications in bioinformatics.
One of these applications is
the problem of finding genes in genomic DNA sequences. A genefinding HMM tries to capture the statistical properties of these special regions of the genome, incorporating these into a probabilistic model that can be used to predict which regions of the genome contain protein-coding genes.
This talk will explore how this is accomplished and look at the results of these efforts.