MacDATA fellows using cutting-edge techniques to analyze data in new ways
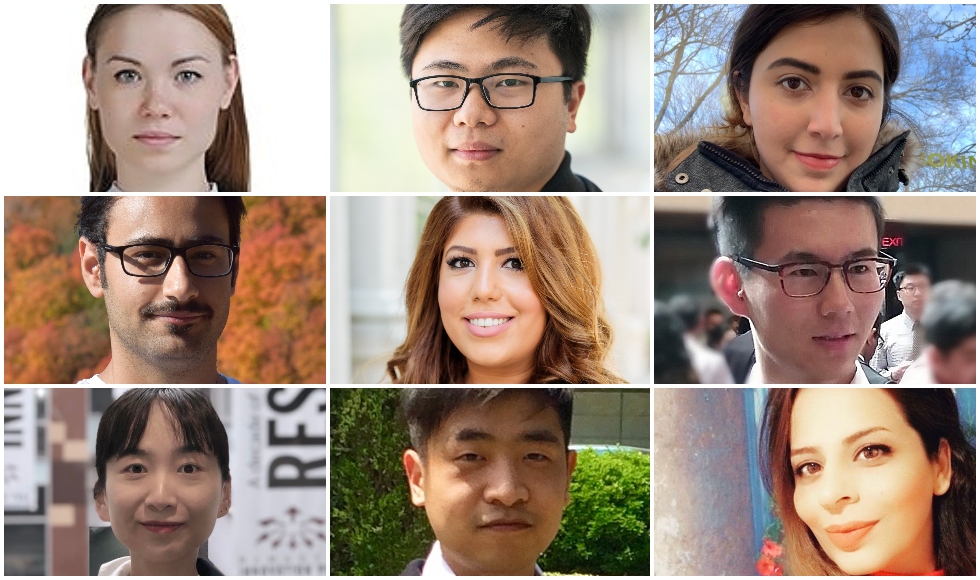
The 2020 MacData Fellows. Top row, from left: Gaisha Orlova, Pengxiao Xhou, Leila Mousapour. Middle row, from left: Iman Sadeghi, Mahdis Kamali, Jianpeng Liu. Bottom row, from left: Yujiao Hao, Jing Wang, Somayeh Ghazalbash.
From using machine learning techniques to predict the health care needs of older adults, to using data analysis approaches to optimize supply chain management, nine McMaster graduate students are harnessing cutting-edge data science techniques to shed new light on these and other research areas.
The students have received fellowships from McMaster’s MacDATA Institute to pursue interdisciplinary projects that apply data analysis, collection and curation methodologies to a number of areas, including mental health, business, and linguistics, among others.
“New and innovative data science methodologies are allowing researchers to get more from their data than ever before,” says Paul McNicholas, director of the MacData Institute and Tier 1 Canada Research Chair in Computational Statistics. “This fellowship brings together students and researchers from all faculties to approach data analysis in novel ways – ways that are helping to reveal new insights from increasingly complex data.”
Each fellow is co-supervised by at least two faculty members who, together, cover data analytics and subject matter expertise. At least one of the supervisors is a member of the MacDATA Institute.
The fellowship program is part of the MacData Institute’s mission to promote innovative research and training programs related to data science, and to work with and support faculties and institutes on data issues and initiatives.
2020 MacDATA Fellowship Projects:
ABLE Project
Yujiao Hao, Computer Science
The Art-based therapies to encourage Longevity in Elderly participants (ABLE) project, led by Humanities and Health Sciences in collaboration with the Engineering, was funded by McMaster Institute for Research on Aging (MIRA). The ABLE project offers arts‐ and games- based movement experiences using its unique unobtrusive wearable technology to encourage older adults to engage in therapeutic exercises. The goal of the fellowship is to provide a means of wearable sensor-based human activity recognition. Supervisors: Paula Gardner, Rong Zheng
Addressing Level of Care Issues in Older Canadians
Somayeh Ghazalbash, Health Policy and Management
This project will use machine learning approaches to develop a data-driven clinical decision support system to predict expected or future health conditions in older adults. This system could inform hospital discharge decisions and help more efficiently deploy scarce health care resources by predicting which patients might need alternative levels of care. Supervisors: Manaf Zargoush, Alexandra Papaioannou
Predicting Contaminants in Wastewater Treatment Plants
Pengxiao Zhou, Civil Engineering
Using a number of different data science approaches, this program will develop a toolkit to predict influent and effluent emerging contaminants at Wastewater Treatment Plants. The knowledge generated by this project could be used by various regions in Canada and internationally, as well as in a number of environmental engineering fields. This research could also help enhance Canadian environmental protection efforts and inform public health policy. Supervisors: Zoe Li, Wenbo He
Non-parametric regression modelling of time-series data
Gaisha Oralova, Cognitive Science of Language
This project aims at finding and validating a technique for analyzing electrophysiological data. The goal is to reveal the earliest time point where the effect of interest begins to influence cognitive processing of a word. Advanced statistical methodology may be suitable for this kind of analysis. Supervisors: Victor Kuperman, James Reilly
The impact of sharing economy rental markets
Iman Sadeghi, Marketing
This research will explore the impact of the sharing economy rental market on the traditional rental market. In particular, this research will examine how different aspects of the short-term rental markets of the sharing economy impact the use and prices of the traditional rental market. Supervisors: Sourav Ray, Matheaus Grasselli
Big Data Solution for WiFi-based Indoor Localization
Jianpeng Liu, Computing & Software
Location Based Services – such as navigation and tracking – are used widely around the world. However, when used indoors, these applications have difficulty providing the same level of positioning accuracy, continuity and reliability as they do outdoors. To resolve this problem, a machine learning model will be built to predict the indoor location of users in real-time based on the received WiFi signal. Supervisors: Paul McNicholas, Rong Zheng
Examining Predictors of Mental Health Service Use among Youth in Ontario
Mahdis Kamali, Health Sciences
The aim of this research is to use machine learning techniques to understand the predictors of mental health service use among youth in Ontario. This study will examine the multifaceted nature of mental health care access by drawing on a range of disciplines including health equity, health systems and quantitative methodology. Supervisors: Paul McNicholas, Kathy Georgiades
Data-Driven Reinforcement Learning for Supply Chain Control
Jing Wang, Computational Science & Engineering
The objective of this research is to investigate the application of reinforcement learning (RL) to optimal decision making in supply chain systems. The goal of the Fellowship work is to investigate the application of RL to the optimal control of supply chain systems. This will include investigation into data requirements and data handling problems for a system of this type. Supervisors: Christopher Swartz, Kai Huang
Optimizing source localization for machine learning pipelines in open-ended non-invasive clinical brain-computer interfaces for communication
Leila Mousapour, Computational Science & Engineering
The specific goal of the research proposed for this MacDATA-supported fellowship is to investigate the impact of mapping electrode-space signals to source space as a way of improving the performance of a brain-computer interface (BCI) system for communication. This work will be in addition to, but also beneficial to, the broader goal of my thesis to develop a BCI communication system for coma patients. Supervisors: Sue Becker, Ranil Sonnadara